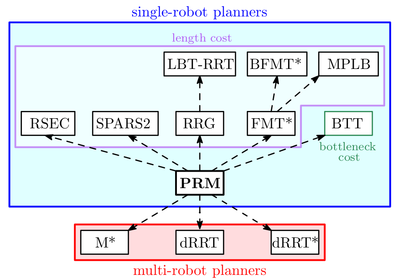
We develop a new analysis of sampling-based motion planning in Euclidean space with uniform random sampling, which significantly improves upon the celebrated result of Karaman and Frazzoli (2011) and subsequent work. Particularly, we prove the existence of a critical connection radius proportional to \(\Theta(n^{-1/d})\) for \(n\) samples and d dimensions: Below this value the planner is guaranteed to fail (similarly shown by the aforementioned work, ibid.). More importantly, for larger radius values the planner is asymptotically (near-)optimal. Furthermore, our analysis yields an explicit lower bound of \(1-O(n^{-1})\) on the probability of success. A practical implication of our work is that asymptotic (near-)optimality is achieved when each sample is connected to only \(\Theta(1)\) neighbors. This is in stark contrast to previous work which requires \(\Theta(\log n)\) connections, that are induced by a radius of order \((\log n / n)^{1/d}\). Our analysis is not restricted to PRM and applies to a variety of “PRM-based” planners, including RRG, FMT* and BTT. Continuum percolation plays an important role in our proofs.